Autonomous vehicles have been driving advances in vision technology, but vendors are targeting other practical applications because broad adoption of self-driving vehicles appears to be taking longer than expected.
Virtual reality (VR) and augmented reality (AR) both have need of improved cameras and sensors for practical use cases, while the trend toward “uncaging robots” for industrial applications such as warehouse fulfillment and manufacturing requires smarter vision technology, which spans 2D cameras to 4D LiDAR.
Layering artificial intelligence (AI), machine learning, and neural networks onto computer vision technologies further expands the many applications for a wide range of sensors and cameras.
Industrial segment is moving fast as automotive
SiLC Technologies CEO Mehdi Asghari said the biggest potential for the company’s 4D plus technology is in the industrial space. “We need collaborative robots.” In an interview with Fierce Electronics, he said many of SiLC’s customers are looking to automate their warehouses with robots because they can’t find enough people to do the work.
Developing advanced vision technology takes investment that needs to be paid off quickly, he said, which is why SiLC isn’t solely focusing on the automotive market due to its long qualification cycles. “The focus for us as a company was industrial and robotics and that has paid off,” he said.
Other applications SiLC is seeing more of are inspection, including remote inspection, as well as construction and surveying, Asghari said.
The “plus” in SiLC’s technology is the ability to add polarization information, he said. The company is also working to provide multi-spectral information.
SiLC customers value the micrometer to millimeter level precision of its Frequency Modulated Continuous Wave (FMCW) LiDAR technology, dubbed Eyeonic, as well as the velocity, Asghari said-- both of which are valuable in industrial robot applications. “It enables them to predict and do hand eye coordination for robots that otherwise would be very hard.”
Asghari said polarization allows for more advanced materials detection, including the ability to see through more transparent material such as bubble wrap. They can also mount as many sensors as they want, he said.
Power consumption and data costs are key considerations
FMCW LiDAR is not a new technology, but it is complicated, Asghari said. “It's a very complex optical instrument and the specifications that you need for the different components are very high.” Traditionally, he said, FMCW has been quite expensive and bulky to deploy, but SiLC is integrating everything into a single photonic chip using mature semiconductor fabrication processes.
Silicon manufacturing offers affordable high-volume scaling of complex devices and technologies, Asghari said. “These are really high-performance optical circuits that we make on a single silicon chip.”
The cost of the chosen vision technology is dependent on many factors, Raj Khattoi, senior director of sensor systems and IoT at Infineon Technologies told Fierce Electronics. Power consumption is one, he said, but so is the cost of processing and moving the collected data. “Cloud storage is not free.”
LiDAR of any kind remains the most expensive of all four broad categories of sensors, which means it’s only used when absolutely needed, such as real time processing for self-driving vehicle cameras, Khattoi said.
A lower power camera can stay on all the time, but that means it’s always collecting data, he noted. “Ultrasonic radar has the biggest advantage because you can basically put a radar behind any plastic or glass or glass devices, so you don't have to change your industrial design,” he said. “It's at a sweet spot of power consumption versus performance.”
On-chip sensors getting smaller
No matter the sensor type, the trend is toward miniaturization, although mechanical sensors have limits to how much they can be miniaturized.
A LiDAR sensor on a chip for automotive applications is now available in packages as small as 5 mm x 10 mm, Khattoi noted, while the smallest radar sensor package is about 3mm x 6 mm including antennas on package, which reduces the overall size of the solution.
While companies like SiLC are focused on industrial applications and others have prioritized automotive, Infineon’s sensor portfolio has prioritized consumer applications from the ground up, Khattoi said, including smartphones. Those companies that started with automotive first and are trying to take their core technology into the consumer segment are struggling with power consumption and form factor challenges.
Infineon’s Xensiv line is a broad portfolio of sensor types that spans, magnetic sensors, current sensors, pressure sensors, acoustic sensors, 3D image sensors and radar sensor monolithic microwave integrated circuits (MMICs). Most recently, the company announced its XENSIV TLI5590-A6W magnetic position sensor designed for industrial and consumer applications such as industrial sensors, human machine interface, medical and multi-purpose cameras.
Khattoi said Infineon is now in a position where it’s able to scale its consumer sensor technology into industrial and automotive environments. The latter requires a variety of different sensor technologies, he said. “Everybody claims that they can do everything with one sensor, and it's not true,” he said.
Because the transition to autonomous vehicles has been slower than expected, automotive sensor opportunities have changed directions. Khattoi said cabin monitoring systems for safety functions using radar are seeing traction because radar can permeate materials – LiDAR requires direct line of sight.
Practical applications abound for advanced computer vision
Another growing segment for sensor technology is “age tech” to monitor elderly people. Infineon’s radar sensors are being deployed in nursing and elder care facilities to detect falls, Khattoi said. Radar works well in scenarios where privacy is important. It can detect if an older person falls in the shower without the need of an actual camera, he said, allowing them to live independently but connected to assistance should they need it.
Radar can do some things LiDAR can’t, as do Time of Flight (ToF) sensors, which have their own sweet spot in VR/AR applications because it offers granularity as well as a favorable power profile, Khattoi said.
Meanwhile, the combination of AI and machine learning algorithms with various sensor technology allows for more intelligence, especially at the edge, Khattoi said. It also opens possibilities for what is called “physical AI,” which uses AI techniques to tackle problems by observing the world through sensors or by modifying the world through actuators.
Ultraleap is combining sensors and haptics to help make interactions between humans and digital worlds more natural. The company is the result of a merger between Ultra Haptics and Leap Motion, which have combined haptics using ultrasonic waves and computer vision technology for hand tracking to improve VR/AR user interfaces.
Ultraleap Vice President of XR Matt Tullis told Fierce Electronics in an interview that the company foresees VR/AR changing the world in many ways for both consumers and businesses. The Ultraleap hand tracking device uses two cameras and some infrared LEDs. The LEDs pulse in sync with the camera framerate, and the cameras send data back to the computer to track a person’s hand – the images of hands provide data for machine learning that is turned into a collection of XYZ coordinates for 26 different points of a person’s hand.
“You can do things like push buttons, you can make gestures and wave, you can have social interactions and you can actually take action,” Tullis said.
Hand tracking is going to be to VR /AR what the touch screen was to mobile phones, he said. “Our goal is to make it as easy as possible to use VR and our devices with your hands,” he said.
These interactive VR abilities support a wide variety of training scenarios, he said, including teaching flight attendants how to do pre- and post-flight safety checks on diverse types of aircraft without the need for actual aircraft. Other scenarios include training for quick service restaurants, Tullis said. “We work with companies to train people how to make milkshakes and fried chicken. Employees can get hurt doing that with fryers and pizza ovens. There's a lot of food waste.”
Ultraleap’s technology provides sensory feedback that allows users to “feel” what they are holding in a virtual scenario. One of the company’s demos is a virtual bonsai tree demo that enables users to care for a virtual bonsai tree with a headset and advanced haptics array. Users can “water” the tree with the array and feel drips of water coming from their fingers or give it “sun” and feel the warmth in their palms.
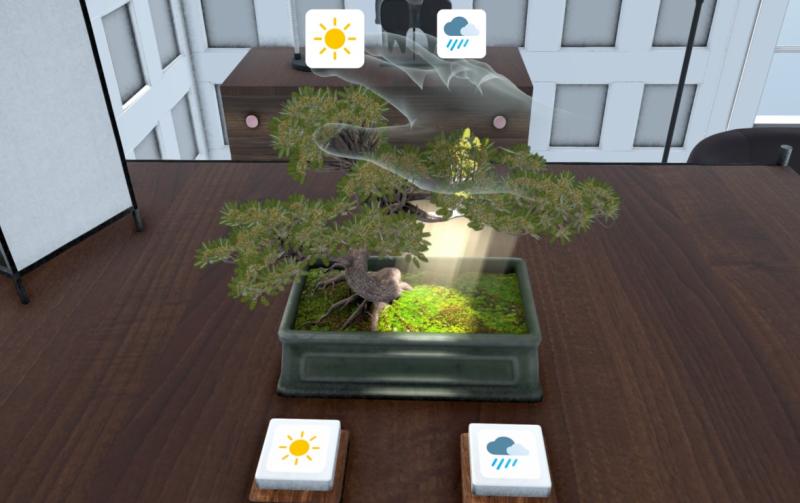
AI expands sensor potential
Tullis noted that VR goes back as far as the 1970s when it was heavily focused on military applications to becoming useful across many enterprise use cases to improve training and do it more cost effectively.
What is different today for AR/VR and other sensor-dependent applications is the availability of AI and machine learning to ingest the data. “We get really crisp, clear images of your hand that we can then feed to our neural network,” Tullis said. “Our neural networks are specifically optimized to see images of your hand and recreate that digitally.”
He said that as the neural networks get smarter it is possible to use cameras that are lower quality and new types of sensors.
Neural networks can learn fast, he said, which allows for highly accurate image classification. “Our network is getting smarter at learning how to recreate a hand regardless of whatever type of image comes in,” Tullis said. “Neural networks now are largely the basis of most of computer vision.”