It is becoming clear that further advances in autonomous vehicles will depend to a significant degree on advances in sensors.
To move forward, the vehicles must improve the reliability with which they identify and classify objects, the accuracy with which they sense the motion of those objects, and, as sensor technology improves, the range of environmental conditions—the operational design domain—in which the autonomy systems can operate. All of these needs depend upon the quality of data coming in from the vehicle’s sensor suite.
That suite has mainly included, for L2 and L2+ autonomy, high-resolution optical cameras, plus radars with limited range and field of view. Short-range sonar or radar sensors have also been used for specific comfort and safety functions such as automated parking, smart cruise control, and lane retention. To reach L3, designers are finding they have to include lidar sensors, which bring a host of disadvantages and limitations, not the least of which is high cost.
But a new generation of 4D imaging radar sensors is changing this picture dramatically. These affordable, high-resolution millimeter-wave radar sensors offer excellent resolution in 4 dimensions: range, azimuth, and—for the first time—elevation, plus accurate, directly-measured velocity. The new sensors also provide longer range and wider field of view, and they support extended operational design domain. All of the data gets delivered in real time to the sensor-fusion processor of the autonomous vehicle.
The conjunction of these features greatly increases the opportunity for increased reliability and wider operating domains. Millimeter-wave radar—unlike cameras or lidar—is inherently able to penetrate difficult weather or lighting conditions. And the combination of high spatial resolution and accurate velocity measurement reduces ambiguities in object detection. The result is more robust object detection and classification over a broader range of environmental conditions.
Radar Evolution
These new sensors are very different from most previous-generation automotive radars. Their advantages are based on mature technologies with years of deployment in military and aerospace applications. Now, increasing integration and performance in semiconductors and antenna fabrication have brought these features down in scale and cost, from a system in an F-18 fighter to a compact module that can be installed at multiple points on a passenger car.
The first significant change has been the availability of low-cost millimeter-wave radar transmitter and receiver hardware, offering good transmitter power and high receiver sensitivity. This in turn enables two more changes: the use of dense multi-antenna arrays—known as multiple-in, multiple-out, or MIMO, in 5G communications—and the use of complicated waveforms that greatly enhance elevation, azimuth, and velocity measurement. Finally, advances in digital signal processing (DSP) intellectual property have made it possible to include the numerical processing power necessary to handle the many channels of high-speed data these sensors generate in real time.
Technical Challenges
Just how these evolutionary changes turn into valuable features is a tale about the interaction of hardware capabilities with firmware functionality. For example, in order to get really high resolution in azimuth and elevation, the sensor must use large-aperture, virtual-array synthesis techniques. Advanced signal-processing algorithms can combine many signals across the time, frequency, or code domains, or some combination of these, to create a virtual antenna array much larger than the physical array.
This greatly increases the resolution: current designs target less than one degree in azimuth, and about one degree in elevation. This resolution produces more uncorrelated measurement points per object, better defining the location and outline of objects than is possible with low-resolution radars. It then becomes far easier for subsequent processing to separate distant objects and to apply machine-learning-based classification algorithms similar to those used with camera or lidar data.
In these systems, design of the transmitted waveforms has a huge influence on the performance of the sensor and the overall cost of the entire solution. Many tradeoffs and considerations become important when selecting the basic waveform structure—the transmitted chirp sequence—creating orthogonality between the transmitted waveform and the process of virtual array creation. For instance, transmitting from several transmitter antennas in parallel will produce numerous virtual-channel measurements on the receiver side. It is necessary to take into account the challenges of separating out those measurements with reasonable algorithmic and processing capabilities.
Selecting an approach to channel multiplexing also involves tradeoffs. Imposing limitations on measurements can result in artifacts. For example, such limitations can induce coupling of angular and doppler measurements, influencing the span of supported unambiguous velocity measurements or creating other measurement ambiguities.
There are also many system level implications to mention. To cite only a few of them: The desire to allow high analog bandwidth and short chirp duration introduces a requirement for higher sampling rates. That in turn makes ADC convertor design harder and increases the ADC cost. Another example: phase based channel-multiplexing schemes require analog phase shifters with high phase resolution. But such phase shifters are a fabrication challenge, and they require delicate and sensitive calibration off and on-line. A third point is that transmitting from several transmit antenna elements concurrently will require more complex thermal design at system level to dissipate the additional heat generated by the transmitters.
Altogether, the design considerations required to exploit high-resolution millimeter-wave radar in a vehicle autonomy system are many and non-trivial. But they are more than rewarded by the increased capabilities these sensors bring to the system.
Real-World Advantages
These capabilities are more than just improved numbers on a data sheet. They are functional differences that can translate into increased safety, autonomy, and operational design domain in real-word vehicles, all while driving in real-world situations.
For example, the increased volume and accuracy of 3D position and velocity data can significantly improve the autonomous vehicle’s ability to identify objects. The better data from the sensor allows the vehicle to make delicate but vital distinctions—separating the strong reflected signal from a large truck from the weaker signal from a nearby small child, for example. Real-time Doppler velocity measurements mean, among other things, the ability to detect a sudden change in an object’s velocity at once, rather than after several scans of the field of view, and the ability to separate close-together objects moving at different velocities.
All of these benefits add up to a better understanding of the situation around the vehicle. And that means greater safety. Add to this the ability of high-resolution millimeter-wave radar to operate in conditions of poor visibility and in cluttered scenes with complicated lighting, and you get a vehicle autonomy system that can function with greater safety and reliability across a wider range of conditions: just the benefit for which the industry is striving.
A flexible, scalable solution
All of these challenges call for a flexible and comprehensive solution for a radar SOC that is both “software defined” and scalable to support advanced radar processing algorithms, Such a platform would including high-performance DSP engines, optimized hardware accelerators for multi-dimension FFT operations, and a dedicated software development kit. For example, CEVA’s SensPro sensor hub architecture delivers this combination with a family of DSP products that offers a range processing capabilities, making it well suited to address wide range of customers use-cases and requirements
The suite of products allows developers to create different flavors or generation of products using different SensPro family members. With its common architecture, simple and smooth migration of DSP software code between the cores preserves investment in the previously developed software codebase and shortens time-to-market.
Of course, the underlying processing capabilities must keep pace with emerging needs, and thus a programmable architecture is required. As market requirements have evolved, CEVA has continuously optimized its SensPro architecture and instruction set to support feature such as:
· Reliable and robust target detection using advanced CFAR schemes (e.g. OS-CFAR);
· Support for enhanced resolution, beyond “Fourier limits”, with super-resolution advanced algorithms;
· Support for inter-frame level processing through processing of radar point clouds to implement advance tracking schemes (for example using Kalman filters and application of a dedicated AI models trained to segment and classify objects from “post-tracker” point cloud)
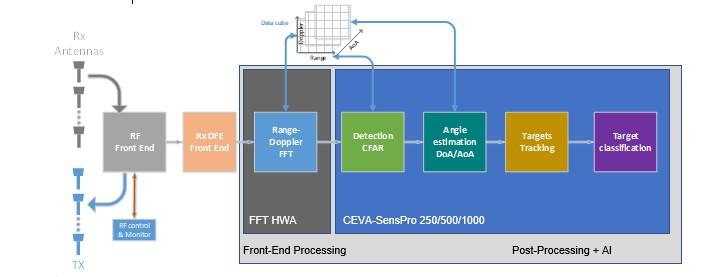
Beyond Driver Assistance
Today’s level of vehicle autonomy, when used responsibly, can significantly improve both vehicle safety and traffic flow. But the ultimate goal remains full autonomy, at least for some categories of vehicles. High-resolution 4D millimeter-wave radar will be a key part of the solution to that challenge. Designers envision a sensor suite that includes a 4D radar at each corner of the vehicle and at least one lidar, all feeding a sophisticated sensor-fusion processor and AI. With sufficient volume and quality of sensor data, adequate processing power for the fusion and AI stages, and sufficient training, it is hoped, errors will be reduced and operational design domain expanded until fully autonomous vehicles, able to operate in nearly any environment, become an accepted part of the transportation system.
But the future will hold more challenges. As the number of vehicles deploying millimeter-wave radar expands, the chances of interference increase. This will force innovation in the waveform processing in the individual radar sensors, and, one hopes, the emergence of standards for vehicular radar. Standards, in turn, could lead to progress in vehicle-to-vehicle and vehicle-to-infrastructure cooperation, which could mean a whole new role for the radar sensors, as parts of a massive distributed intelligence network.
The current lack of agreed-upon standards in automotive radar transmission requires a solution to have a high level of programmability to adapt. This could include, for example, being able to implement possible interference mitigation on a single sensor level, or the addition of coordination mechanisms between the sensors and the infrastructure, so sensors could utilize the resources of the shared spectrum in a safe and efficient way. Continuing progress in underlying semiconductor technology, antenna design, and algorithm development will also keep pace with these emerging ideas.
And the self-driving passenger car is far from the only application for these new sensors. Obviously, there are many other types of vehicles that can benefit from autonomy or advanced driver assistance over a wide operational design domain, particularly as the size and cost of the sensors declines. But the benefits are equally important for stationary applications, such as traffic flow management and pedestrian safety systems in congested areas. One can imagine cooperative systems in which trucks, cars, motorcycles, bicycles, and pedestrians are in continuous communications about their location, velocity, and what they see around them.
Basically, high-resolution millimeter-wave radar sensors will have applications in any situation where understanding a dynamic environment is important and visual camera data is insufficient. Their technology has more than enough headroom to grow to meet new requirements. The limit to their application may be only the creativity of system designers.
Zeev Kaplan is a Product Director in CEVA's Mobile Broadband business units and has been in the communication industry for more than 15 years.
He joined CEVA in 2011 and was a key player in design and implementation of CEVA Cellular Communication products portfolio spanning from CEVA-XC cores to PentaG HW accelerators. He has been granted several patents in the field of communications and signal processing.and holds advanced degrees from Technion, Israel Institute of Technology in Electrical Engineering.