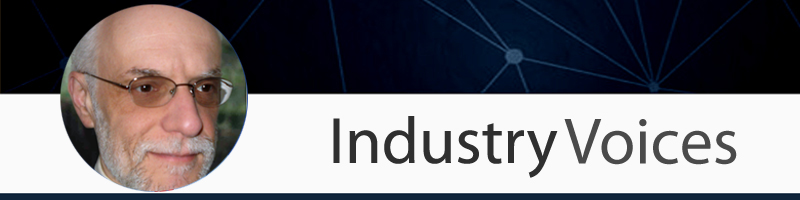
AI is not a “one size fits all” technology, and there is no “one size fits all” processor for AI. There are a plethora of models being developed for various purposes.
And while Large Language Models (LLMs) like ChatGPT have garnered many of the headlines, AI workloads are being deployed within small devices like smart IoT, into clients like smartphones and PCs, at the edge like vehicles, and into large datacenters and cloud infrastructure. And while many headlines have been grabbed by the largest of these processors, mostly from Nvidia, there is a vast continuum of processor providers that will be performing AI workloads across an entire ecosystem of devices and applications. The figure below is a simplified representation of the market for AI processors.
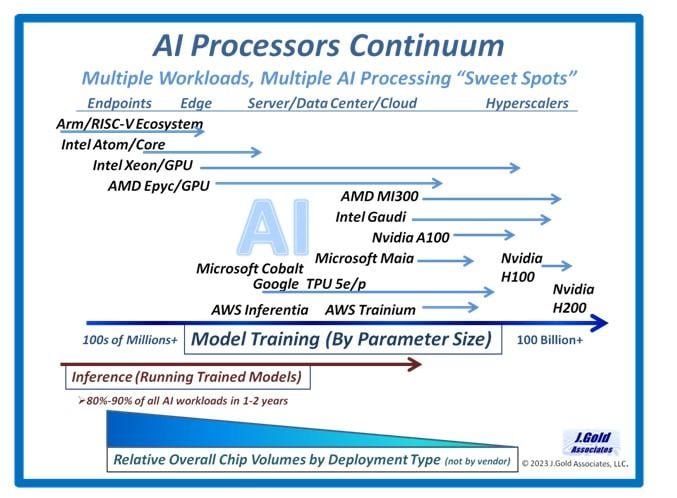
Training vs Inference
Much of the work done on the very largest devices based on massively parallel processing and often running hundreds or thousands of high-power GPU/NPU accelerated processing elements, is being performed on model training – taking vast amounts of data points, or parameters, and building a model based on potentially billions or hundreds of billions of parameters. But at the end of the day, these massively trained models need to be deployed into production settings, while inference processing, which is much less intensive, is used in the analysis of specific applications run against these models. As a result, we expect that in one to two years, we’ll see 80 to 90 percent of all workloads being run on inference processing, and on a variety of chips from relatively small processors in end-user devices, to mid-size processors running at the edge or in the data center.
Multiple AI sweet spots powered by multiple vendors
We expect a huge ecosystem of AI-accelerated processing to be available in the next one to two years, and from a large number of vendors. At the lower end, we’re seeing ARM and RISC-V adding AI acceleration capabilities to their respective ecosystems that will power a variety of smart devices. Processors from Qualcomm and MediaTek, often powering the premium brand of smartphones (such as the latest Samsung devices), already have NPUs built in for advanced video, photo and audio/text AI-based processing. Large end point devices, like PCs, will also have AI accelerators built into chips from Intel and AMD that will add a host of new AI assisted features to the devices themselves and the apps that run on them, and including new OS features being built into Windows.
Edge computing will add AI acceleration to bring low latency and increased security/privacy to a distributed network of close to the end user solutions. And data centers will increasingly see chips from Intel and AMD, along with other suppliers, adding AI capabilities in both inference and training to standard platforms running a variety of typical enterprise applications. In the public cloud area, we’ll continue to see high end solutions powered by Nvidia, Intel Gaudi, AMD, and potentially others, but increasingly we’ll see the hyperscalers (AWS, Microsoft Azure, Google Cloud) develop their own optimized AI chip solutions to enhance operations inside their infrastructure and provide their customers with lower cost and potentially more operationally-focused options. We expect the number of processors with AI acceleration built-in to become the majority of the market in the short term, just as the industry transitioned to integrated GPUs in the past as they became mission critical.
Where are the volumes?
From a dollar perspective, the high end of the market is driving sales figures in the short term. But longer term, we expect the vast majority of AI-enabled chips to be in the mid to lower end of processing, where specialized AI acceleration provides the ability to do relatively simpler model training and especially in the ability to run the vast amounts of trained models that inference is optimized for. There is no way to know exactly how the volumes will play out longer term, but we expect a similar dynamic to that of the GPU, where integrated GPUs far surpass the volumes of standalone GPUs used for only the most difficult tasks needing the highest performance, and by users not necessarily interested in the cost.
Winners in the volume race
With its dominance in the x86 solutions and open source platform that runs from server to cloud to hyperscaler, Intel stands to benefit greatly from the shift. It already enables many of its processors with AI acceleration, even now extending to its traditional dominance in the PC market with Intel Core. And since it has a large share of the server market, it stands to benefit here too as it already adds AI acceleration NPUs to its Xeon family. AMD is also adding AI acceleration to its processor families to compete in the new AI world, and is challenging Intel for performance leadership in some areas. And Apple is adding AI capability to its proprietary chips running the Mac, iPad and iPhone. We expect that at least 65 to 75 percent of PCs will have AI acceleration built-in in the next three years, as well as virtually all mid-level to premium smartphones.
In the server, datacenter and cloud space, the move to AI means a replacement market is emerging that should help lift all processer providers. This will fuel a refresh cycle over the next two to three years that should be substantial. And while AI acceleration takes a lot of the hype, there is also a major requirement for high-speed, high-performance interconnect and network, as well as new high bandwidth memory to keep up with the advanced workloads. This is a major opportunity for connectivity processor providers like MediaTek and others, as well as high performance memory producers like Micron, Samsung, etc.
Bottom Line: AI is rapidly expanding from its current base of high-performance chips running massive training systems to encompass a wide range of inference-based solutions. This expansion will enable a wide array of new solutions built on the acceleration capabilities inherent in the majority of next generation chips. The AI Continuum will provide a way for nearly every chip maker to expand market share, while app vendors find new ways to enhance their solutions. As with many markets in the past, while the high end may lead the onslaught, the real volumes will migrate to the everyday workload processors, and this will happen in the next one to two years.
Jack Gold is founder and principal analyst at J.Gold Associates, LLC. With more than 45 years of experience in the computer and electronics industries, and as an industry analyst for more than 25 years, he covers the many aspects of business and consumer computing and emerging technologies. Follow him on Twitter @jckgld or LinkedIn at https://www.linkedin.com/in/jckgld.