After the recent crash of a Tesla car due to the inability to distinguish a foreground and background of same color, a need has arisen to improve discrimination of objects of a similar color to aid autopilot systems. This project looks at four methods of distinguishing a foreground and background of the same visible color: Texture, Spectral Analysis, Polarization, and Infrared Imaging.
Texture
It is possible to detect texture differences between objects of the same color by image processing to detect light and shadow caused by texture. A visible spectrum camera was used to capture differences between surfaces and image analysis was done to look for differences in the reflected light. In the experimental setup of figure 1, a single line was digitized to show the textured component as shown in figure 2. The rough area is clearly distinguishable from a smooth area with brightness variations of about 15%. This method is viable; however, it relies on the presence of texture which may not always be the case.
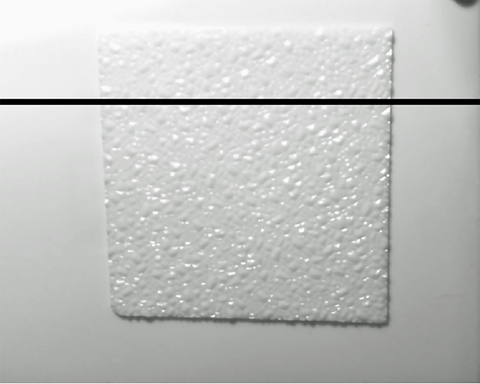
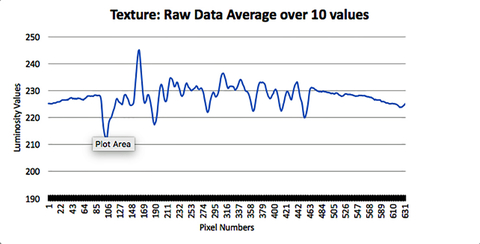
Analysis Of Color Spectral Differences
It is possible to create the same color by mixing different colors. For example, in color light sources, mixing red, green and blue will create white or mixing blue and yellow will also create white. In a standard color chromaticity chart as in figure 3, any two or more color sources will mix to give a color that is at the centroid of the colors. In a similar manner reflected colors that appear to be the same color may have different spectra.
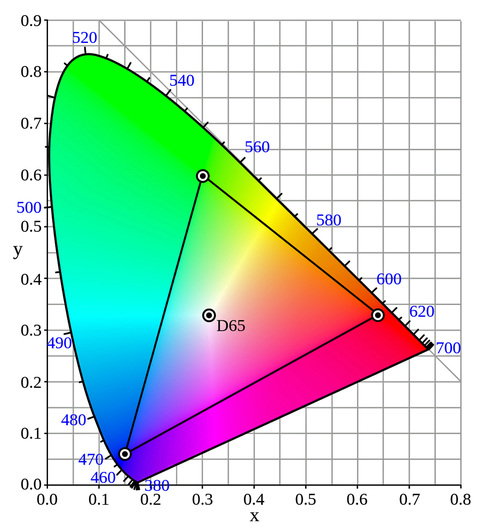
Analysis of the same color of paint from two different paint manufacturers by analysis of their RGB values from a camera produced some differences, however it was unclear if this was due to the original colors being slightly different or if it was due to the same color being represented by different pigments. Accurate results would require analysis of the full spectrum, by means of a prism or diffraction grating, rather than an interpretation of RGB, which is itself an interpretation of non RGB colors by a camera. This method was abandoned due to the lack of availability of precision equipment required to study it.
Polarization
It is well known that light from the sky can become polarized due to, for example, Rayleigh scattering. It is also well known that reflections from non-metallic surfaces can polarize light depending on the angle of incidence as shown in figure 4. Viewing a white object against a white sky may look very different when viewed through a polarizing filter. An experiment was done to look at a white paint swatch against a white sky and to photograph it with a polarizing filter. Results were mixed with some contrast enhancement, which depended critically on the part of the sky that was in the background. This result is expected because Rayleigh scattering is dependent on the direction of incidence of the light. This method can create contrast, however it cannot always be relied upon due to the variability off conditions.
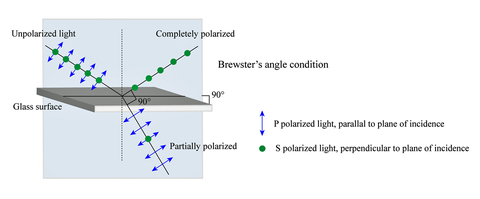
Near Infrared Imaging
The final method evaluated was the infrared method. The original thought was that objects of the same visible color would look different in infrared light, and such objects could be discriminated by examination of the infrared image.
A standard webcam was modified to see near IR by removing the IR filter from the lens stack and replacing it with an exposed developed color negative film to block visible light. (This method of creating a near IR camera is well documented on the internet).
Paints of the same approximate visible color from two different manufacturers were tested to see if there was any difference between the infrared reflectivity and the visible spectrum reflectivity. It was found that there is a difference in the intensity of infrared reflectivity between surfaces, which appeared to be of the same color in visible light. These differences could be used by an object detection algorithm, fed by both the infrared and visible images simultaneously.
Infrared Transmissive Paints
During the testing of the IR method, it was realized that some paints were mildly transmissive to IR and the background material on which the paint was sprayed had some bearing on the reflectivity in the infrared spectrum. This discovery led to the idea of placing an infrared reflective pattern behind the (visible light reflecting) paint. During further investigation it was found that colored plastic sheets reflected visible light but were transparent to infrared light. Colored plastic file covers were found to work quite well. As shown in Fig 5, a layer of colored plastic sheet measuring 0.015-in. thick was placed in front of a photocopy of a checkerboard pattern on standard paper from a laser copier.
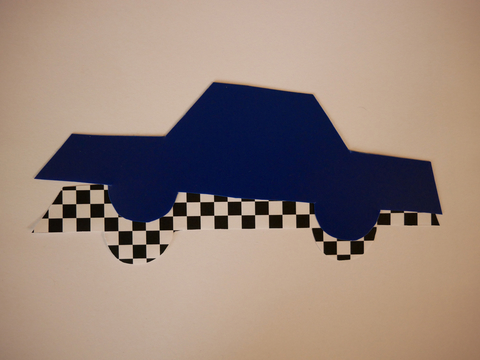
This method of a layer of visible light reflecting pigment backed by a layer of infrared reflective pigment with a pattern was found to be a highly effective, robust method of creating contrast between two objects of the same visible color. As seen in figure 6, which shows the visible image, and figure 7, which shows the same image under infrared light.
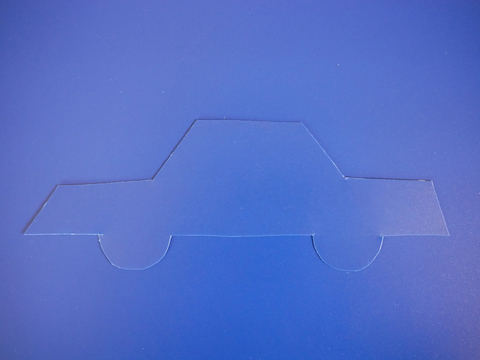
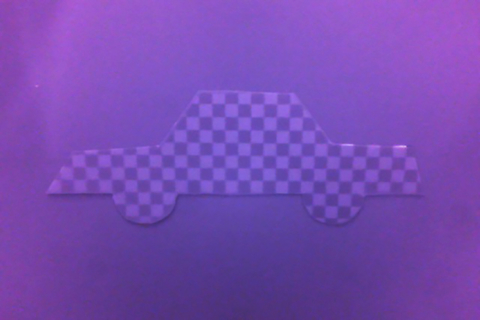
Further investigation of this novel method revealed that it works quite well in daylight which contains infrared light, however it was found that artificial lighting indoors did not always emit infrared, and IR emitting LEDs were required to illuminate the scene. Similarly, in a real autopilot application, illumination from a source like a headlight would be required at night.
While a checkerboard pattern used in the tests created a distinct signal as seen in Fig 8, it may be possible to paint an infrared pattern like a barcode on each side of a vehicle. This would not only identify the exact type of vehicle but also identify the side of the vehicle which is being viewed, allowing any autopilot algorithm to react to a situation much faster and with more assurance than with a system which attempts to recognize an object by image analysis.
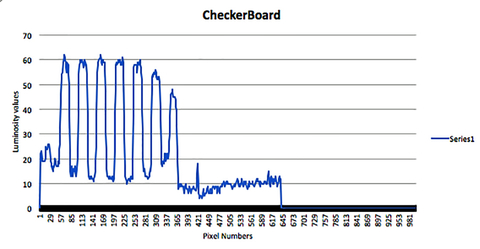
In conclusion machines need not discern objects by detecting silhouettes or shape recognition algorithms, which mimic methods used by the human eye and brain. Instead, they can rely on rich machine-readable messages hidden in plain sight.
About the author
Rohan Karunaratne attends Stratford School in Fremont, CA. This article summarizes his science project "Improving Car Autopilots" which won 1st place in the category of Robotics and Intelligent Machines at the Alameda County Science Fair in Silicon Valley. He can be reached at [email protected].