Nvidia’s introduction of the Blackwell accelerator chip at GTC2024 raised again the vital questions of what it costs enterprises to pay in dollars for hardware to stay ahead in the GenAI revolution and what it costs to pay for energy--assuming there’s enough electricity available to meet demand.
Energy and hardware costs are arguably the two biggest concerns on the minds of data center architects everywhere, but hyperscalers and big data centers want to compete or even lead in the AI space, which means CEOs are hyperfocused on GenAI growth, almost willing to accept whatever the cost. It is complex to decipher up-front costs for chips and servers, when the TCO is factored in alongside what the local utility is charging for electricity. Top-flight enterprises analyze (hopefully) what revenues can be obtained with a new, yet-to-be-invented GenAI application for sale, or how GenAI will lower costs for accountants and coders, among other work groups.
Even so, Nvidia argues it is dealing with rising costs for hardware and the need to reduce the sucking sound (or the buzzing sound) of electricity coming from the grid. (Nvidia argues it is also addressing security at the data center level.)
What it will cost
Nvidia CEO Jensen Huang told CNBC’s Jim Cramer that a Blackwell chip will cost maybe $30,000 to $40,000, but seemed to hedge on that amount later when meeting with reporters at GTC. Dylan Patel, chief analyst at SemiAnalysis, described the base cost of a B100 chip at $30,000, scaling up to $37,000 in comments to Fierce Electronics.
SemiAnalysis also wrote, maybe almost tongue-in-cheek, that the “B” in Blackwell could stand for “Benevolence” because previous generation H100s have been tres cher, to say the least. In fact, some data center managers have told Fierce Electronics of paying $60,000 to $80,000 for a single H100, so maybe Nvidia really is being benevolent in the Blackwell generation. (H100s were also in short supply last year which helped boost the cost and in some cases forced GenAI architects to rent time on H100s from hyperscalers.)
“The pricing will come as a surprise to many, and as such we like to say the B stands for Benevolence, not Blackwell, because the graciousness of our lord and savior Jensen Huang is smiling upon the world, particularly the GPU-poor,” SemiAnalysis wrote.
“Nvidia is on top of the world,” SemiAnalysis continued. “They have supreme pricing power right now, despite hyperscaler silicon ramping. Everyone simply has to take what Nvidia is feeding them with a silver spoon. The number one example of this is with the H100, which has a gross margin exceeding 85%. The advantage in performance and TCO continues to hold true because the B100 curb stomps the [AMD] MI300X, [Intel] Gaudi 3 and internal hyperscaler chips beside the Google TPU.”
Patel added in commments, however, that $30,000 for Blackwell is actually “cheap, relatively” and is above the going H100 price, “but not that much more.”
This Blackwell pricetag, of course, matters greatly when weighing the cost of powering up multiple Blackwells across a big data center, some with many thousands of the GPUs working in concert with costly NVLink connections, liquid cooling and more. Nvidia unveiled the DGX SuperPod AI supercomputer powered by GB200 Grace Blackwell Superchips (that’s the older Grace CPU with the brand new Blackwell) for processing trillion-parameter models for GenAI training and inference.
Each DGX GB200 rack system features 36 Nvidia GB200 Superchips, made up of 36 Nvidia Grace CPUs and 72 Nvidia Blackwell GPUs, connected as a single supercomputer with fifth-generation Nvidia NV Link. The SuperPOD provides 11.5 exaFLOPs of AI compute at FP4 precision and 240 terabytes of fast memory. The GB200 superchip delivers up to 30 times the performance of the Nvidia H100 Tensor Core GPUs for LLM inference.
Each DGX GB200 server rack has 72 Blackwell GPUs, which would alone be $2.1 million at the abovementioned $30,000 price, but that would only be a fraction of the cost. It will ship later in 2024, and pricing has not been announced.
Nvidia said each DGX SuperPOD features eight or more DGX BG200 systems, and can scale to “tens of thousands” of GB200 Superchips connected via Quantum InfiniBand. Customers can deploy a config that connects 576 Blackwell GPUs in eight GB200 systems connected via NVLink. At $30k each, that’s $17.3 million for just the Blackwells inside, but the Nvidia DGX magic is far more than the Blackwells alone. It is not hard to imagine a price all-in for eight GB200 systems into many millions of dollars, but discounting for partners comes into play.
On its own, a single DGX GB200 NVL72 server rack put on display at GTC is a 120-kilowatt system weighing 3,000 pounds to provide 1.4 exaFLOPS performance for up to 27 trillion parameter training workloads. That would be 72 GPUs and 36 CPUs, which Patel priced at “up to $3 million.” ( Again, Nvidia has not yet disclosed pricing.)
The power question
DGX GB200 NVL72 has liquid cooling and 1.44 exaFLOPS of FP4, 13.5 TB of HBM3e and two miles of NVLink cables, all in copper with a white insulation coating. Nvidia picked copper over optical fiber because optical transceivers for optics would have added 20kW to the power draw, Charlie Boyle, vice president of DGX for Nvidia, explained to reporters.
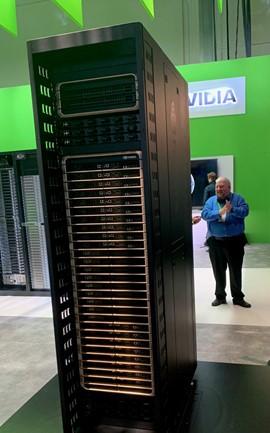
In other words, Nvidia is indeed worried about power. With 120kW of compute, the rack is potentially very hot and Huang said in his keynote it will be liquid cooled with coolant pumped through at 2 liters per second, entering at 25C and exiting at 45C. (Tobias Mann is a master at getting into these numbers at The Register.)
The rundown on the Blackwell platform has confused some people, since Huang said Blackwell isn’t a GPU, but an entire platform, although Nvidia has made it clear the platform has a range of products that are still based on GPUs. There are at least three variants, with the top-end B200 designed to handle 1200 watts, which is 500 watts above the Hopper GPU at 700 watts. The GB200 Superchip platform runs two B200 GPUs and a Grace CPU for up to 2700 watts total.
There is also the Blackwell B200 for DGX and HGX with is optimized around 1000 watts and then the Blackwell B100, which is tuned to 700 watts. The Blackwell GPU is also being incorporated in RTX and AI platforms for Drive Thor and the future GeForce, but some of those power designs are not known.
Blackwell is “designed to very performant and very energy efficient,” Huang told reporters. In an example of training 1.8 trillion parameters in 90 days, Nvidia was able to reduce 15 megawatts in a prior generation down to 4 megawatts with Blackwell. “In 90 days, the amount of input is 4 megawatts, saving lots and lots of energy, [and] saving lots and lots of money, of course,” he added.
Asked by a reporter how Nvidia thinks of designing AI platforms like Blackwell from a power draw perspective, Huang elaborated, “We have to figure out what our physical limits are and take it as far as we can to those physical limits while going beyond. The way you go beyond physical limits it to make things more energy efficient. So the first thing we do is we make things way more energy efficient.” He again brought up the 90 day example of training with GPT-4 with 8,000 older Hopper GPUs over 90 days, compared to 2,000 Blackwells over that period with 4 megawatts, down 11 megawatts over that same time. Blackwell is “way more energy efficient, and because we’re way more energy efficient, then we can also push the limits. Energy efficiency and cost efficiency are job one.”
Blackwell can also generate tokens for LLM models 30 times faster than before, he added. “Nothing on earth became 30 times faster, so the fact we make it 30 times faster says we saved a lot of energy in doing so—30 times less energy to produce the same token. Yep, energy efficiency and cost efficiency are in fact at the core of everything we do. It’s actually first.”
RELATED: Nvidia’s robotic Project GROOT pairs Apptronik humanoid with gen AI